The Client / Condé Nast / Style.com
“We want style.com to rival Net-a-porter and other luxury online platforms”
Through iconic publications like Vogue, GQ and Condé Nast Traveller Condé Nast (CN) has a long history of curating commercial opportunities within editorial pages. The acquisition of renowned fashion site Style.com presented the opportunity to bring the same expertise to luxury fashion ecommerce.
(Project: London, 2015)
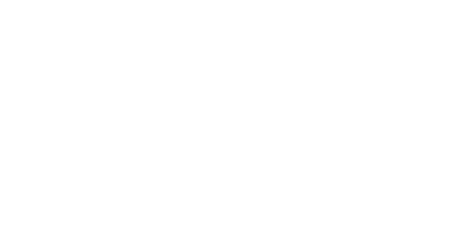
The Challenge
“Can we bring Condé Nast famous editorial insight to online shopping?”
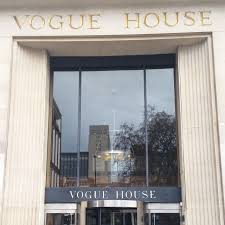
Unlike the hundreds of other shopping platforms that show thousands of filterable products CN could differentiate itself in the market by using the expertise of its editorial staff.
However, the number of curated pages would be immense and need to be constantly updated. Using machine learning was there a way to understand the thinking process behind how editors created product spreads in order to automate the process?
Research
“What decisions go into designing a product spread?”
Working with the editors we ran workshops to understand their thinking process as a linear map of activities, editorial inputs, needs, and signifiers of success.
Looking at what machine learning could improve we started to map ways in which those could enhance a product list page.
Whatever solution we found we had to understand how product sourcing would occur and what the implications that would have on how we displayed content for the end-user and info for the editor/Machine Learning System (MLS).
In terms of baselining our approach we looked into CMS based page builders to see how other companies had approached a visual orientated CMS.
Activities & Outputs
User interviews, departmental workshops, gathering usage analytics, user journey mapping, content mapping, content analysis, information architecture, technical reviews, design review, competitor & market analysis, academic research into search psychology and behaviour.
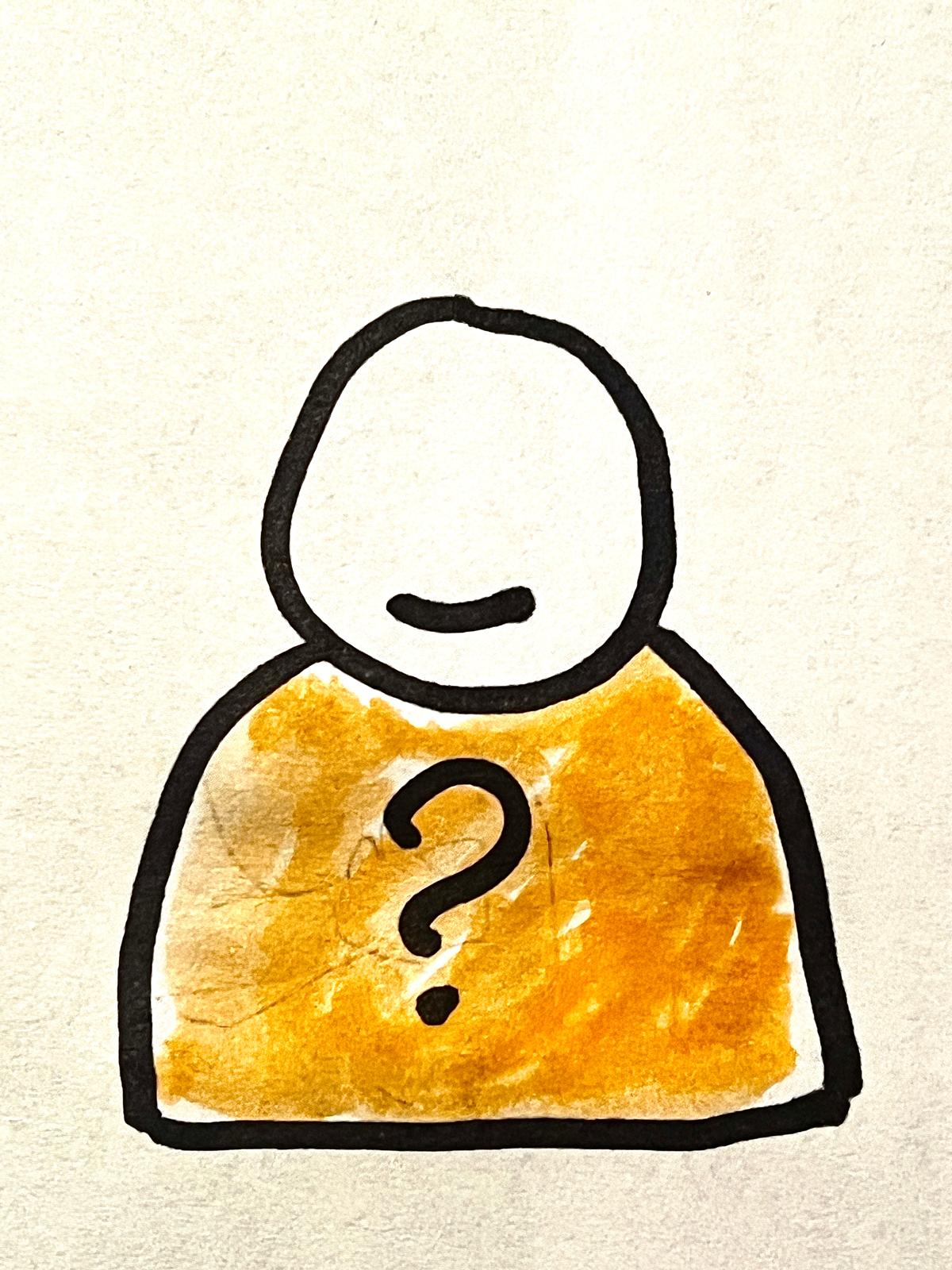
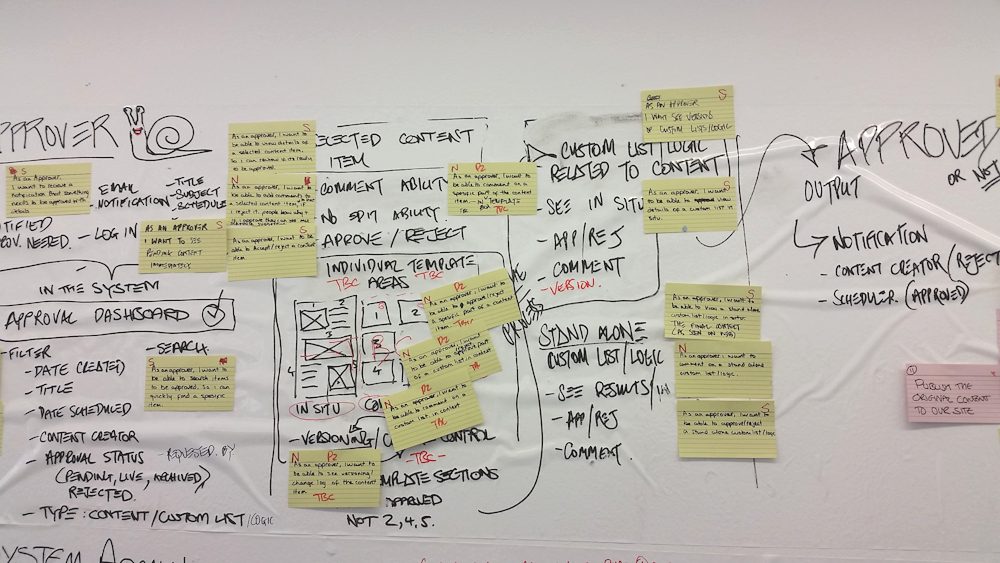
Analysis
“Which processes could be automated? What still relies on editorial oversight?”
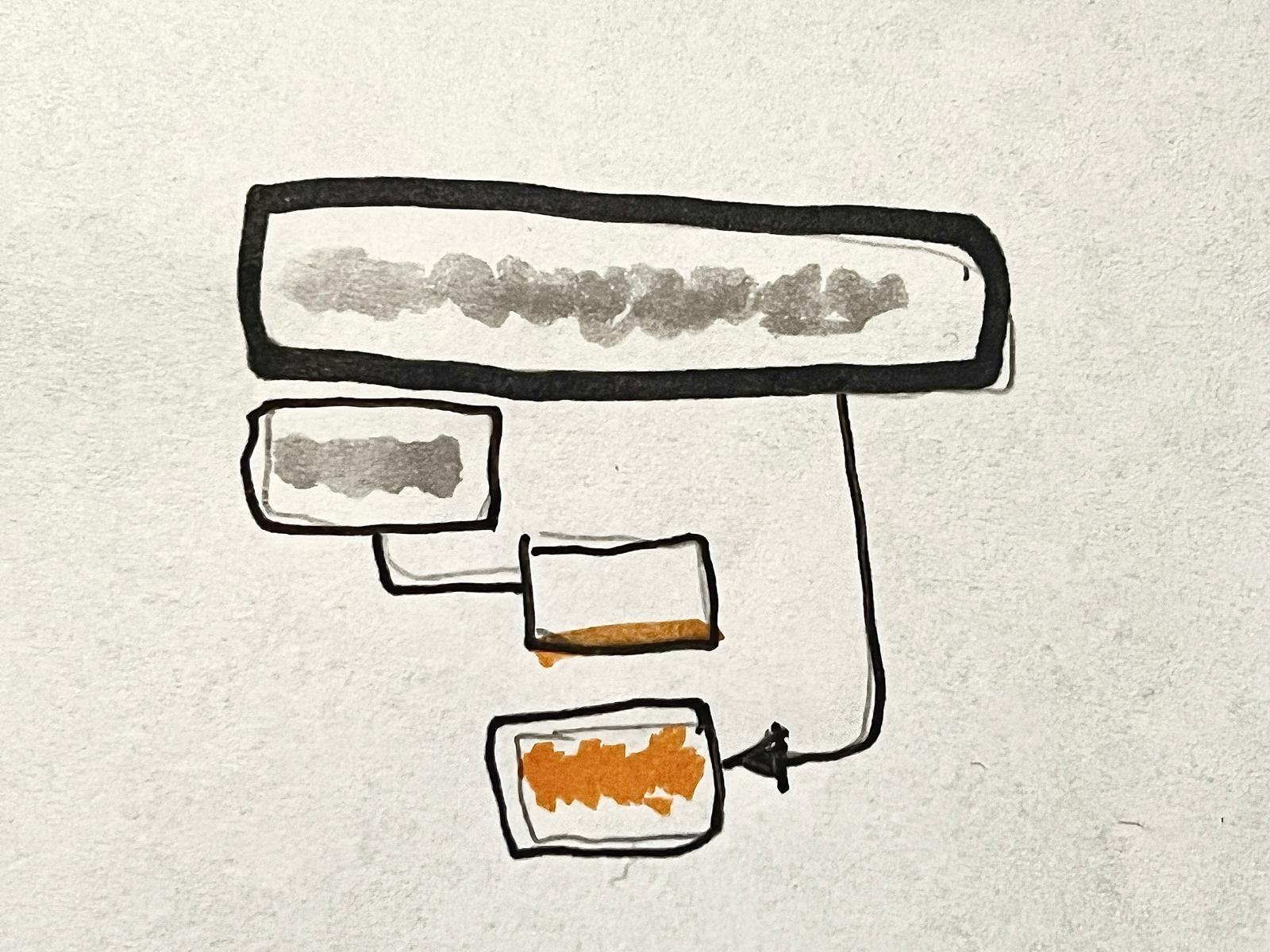
With the ‘as’-is’ editorial journey mapped we came up with a journey simplified and refined ‘golden path’ journey which would make it quicker to curate product look pages.
Many of the decisions editors made to create a layout could be translated to business logic which would inform the layout of related products on the page by the MLS. With a starting list of parameters we recognised the need to add/edit these processes.
Mirroring the publication layout process for page creation a drag and drop UI was found to align most closely with editor needs.
Activities & Outputs
Golden path user journeys and gap analysis, user archetypes, role definition, departmental need definition, technical ecosystem map, content ecosystem map, skeleton IA, problem definition statement, research response matrix (hi-lo severity, hi-lo importance) and design mood-board and workshops to validate analysis with users and stakeholders.
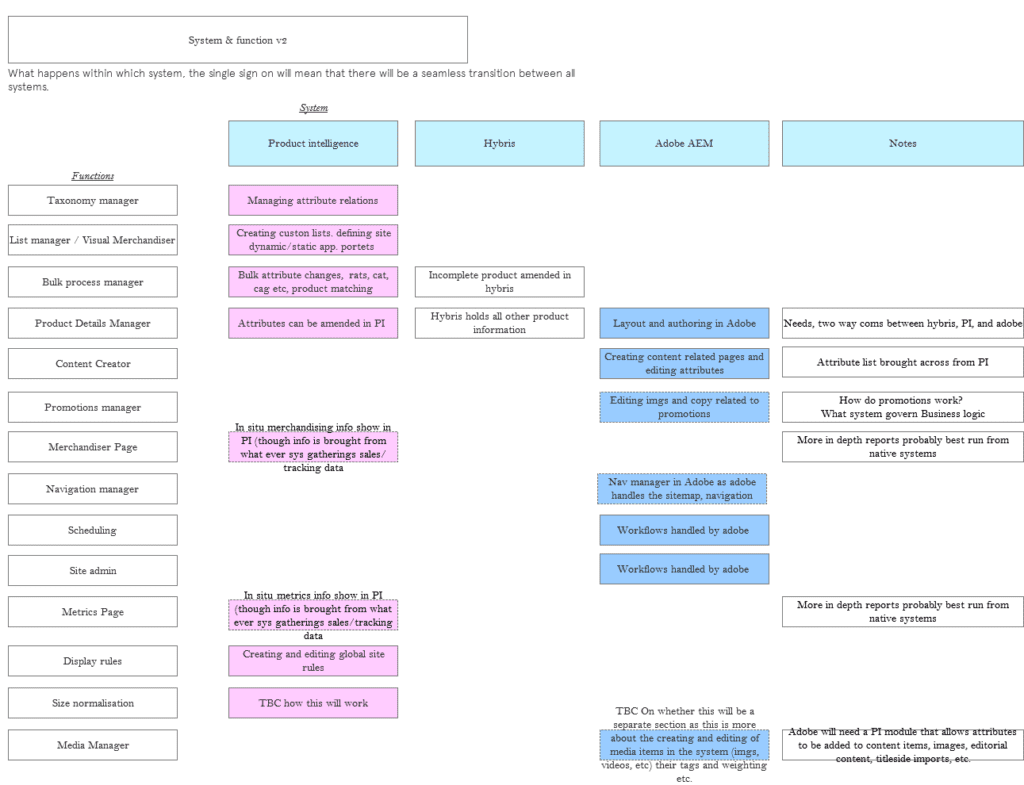
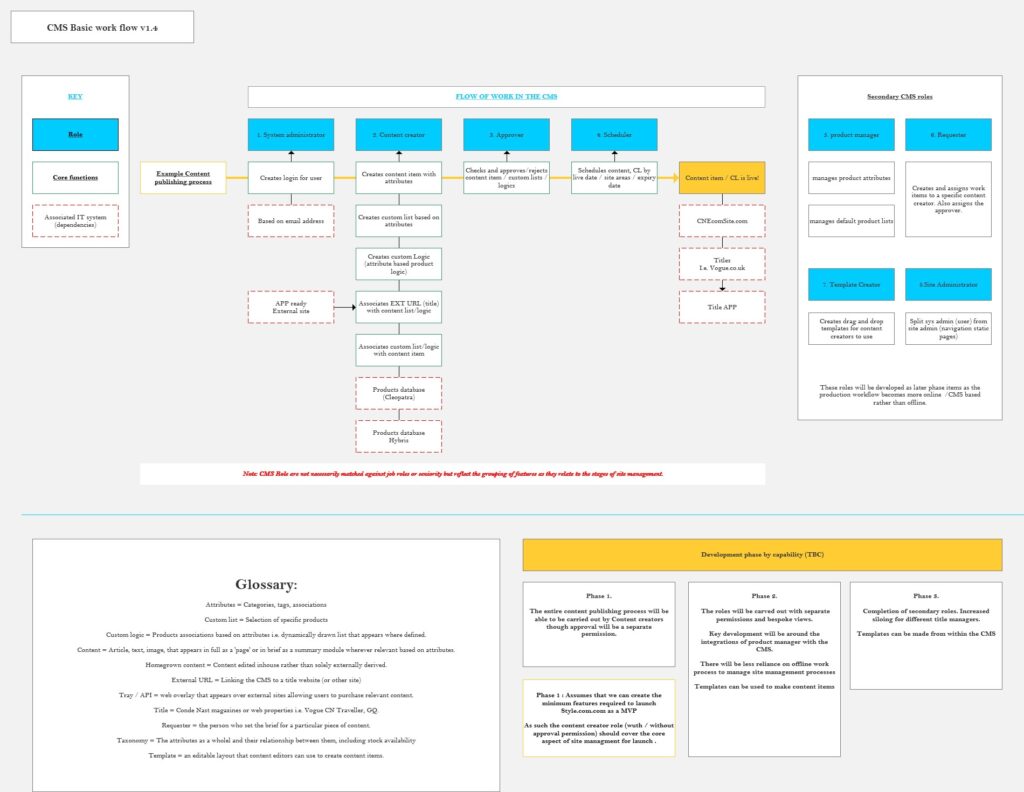
Solutions
“Editors want to check visually rather than leave it completely up automation”
With a list of user journeys required we started ideate solutions around creating a WYSIWYG experience with tools available to tweak related product placements. Editorial inputs such as an article (e.g. houndstooth looks for winter) or photo (e.g. get the James Dean look) could be used as a ‘prompt’ for the MLS to create the page.
After the initial MLS generated page further editorial changes would be ‘read’ by the MLS and associated business logic could then be updated. This would mean that the rules that governed the creation of a page layout could be learnt by the MLS which would then lead to better automation.
The idea being that editors would then only have to schedule and approve pages generated from flagged product focused CN print content (managed by the CN print CMS, Adobe EM).
Activities & Outputs
Solution ideation workshops, wireframes, prototypes, user testing of solution flows, user testing of page layout, delivery workshops with technical teams, liaise with global brand leads on design, design library integration, interaction UI design, cross-departmental review, risks and issues log, alpha and beta user group definition, senior stakeholder presentation and strategic delivery sessions.
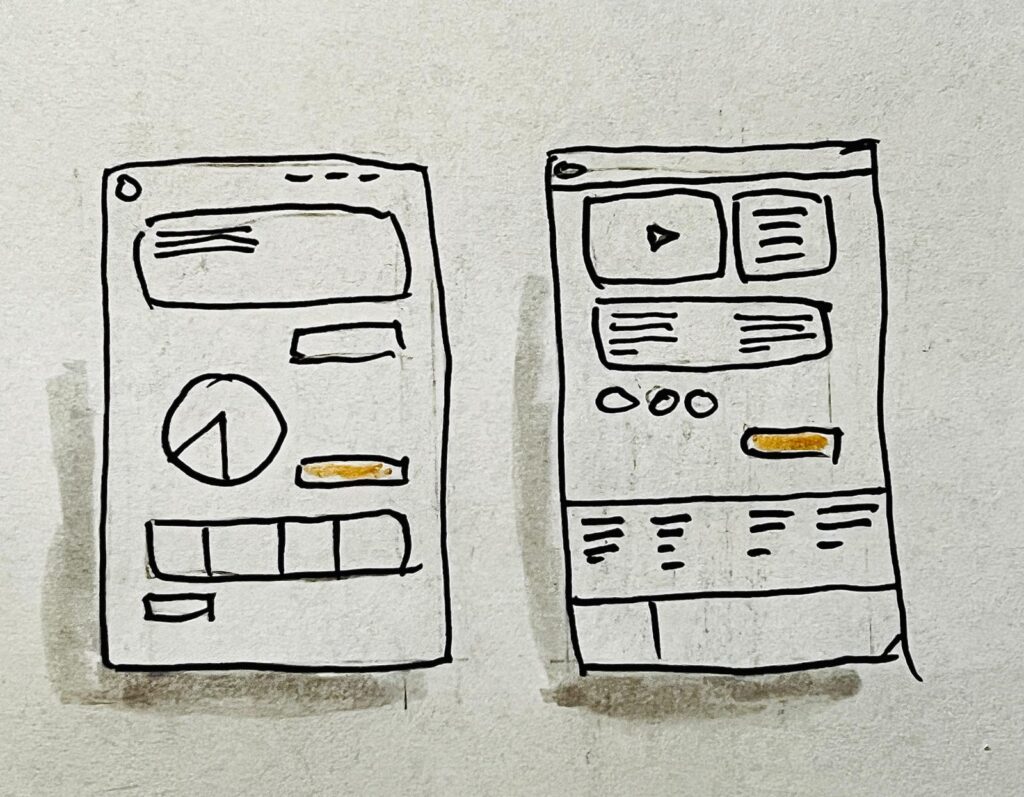
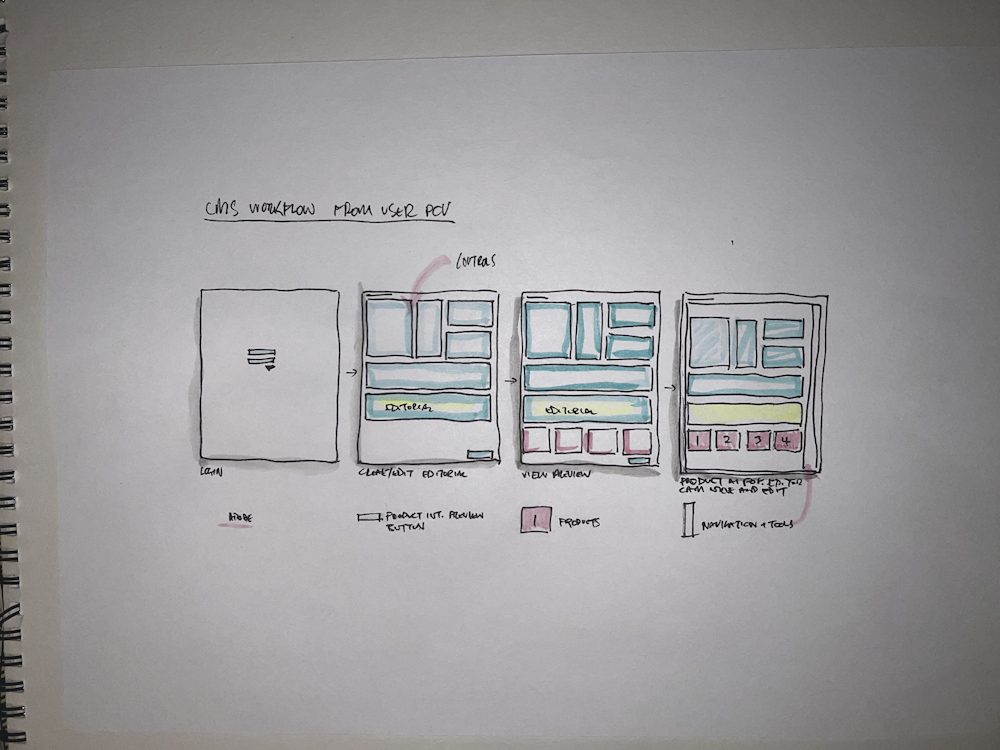
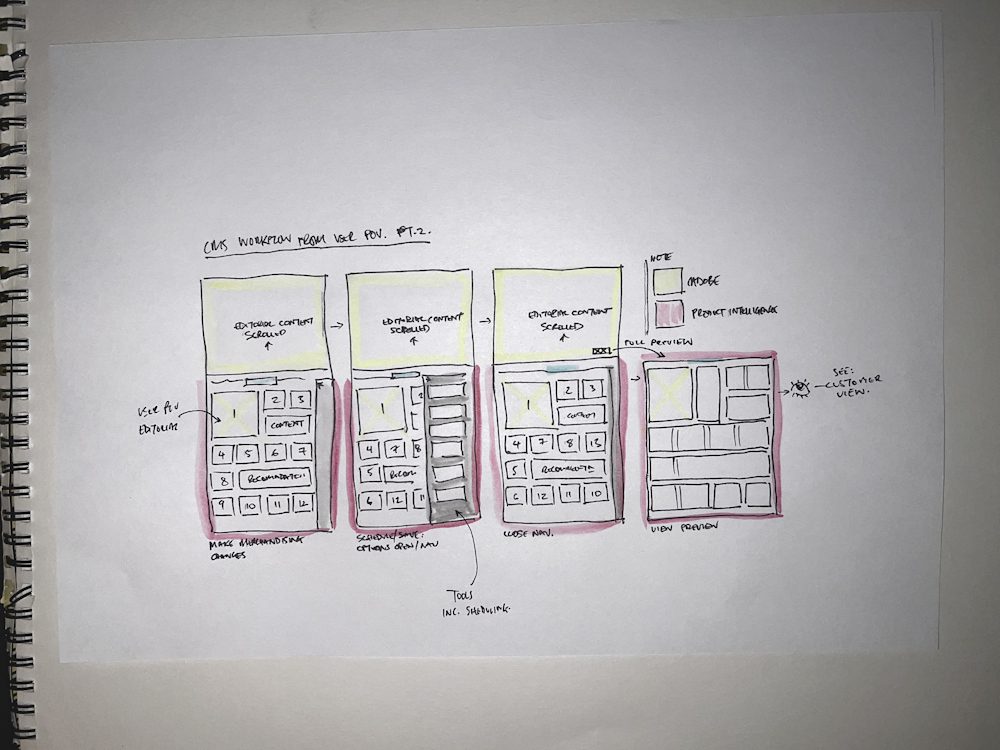
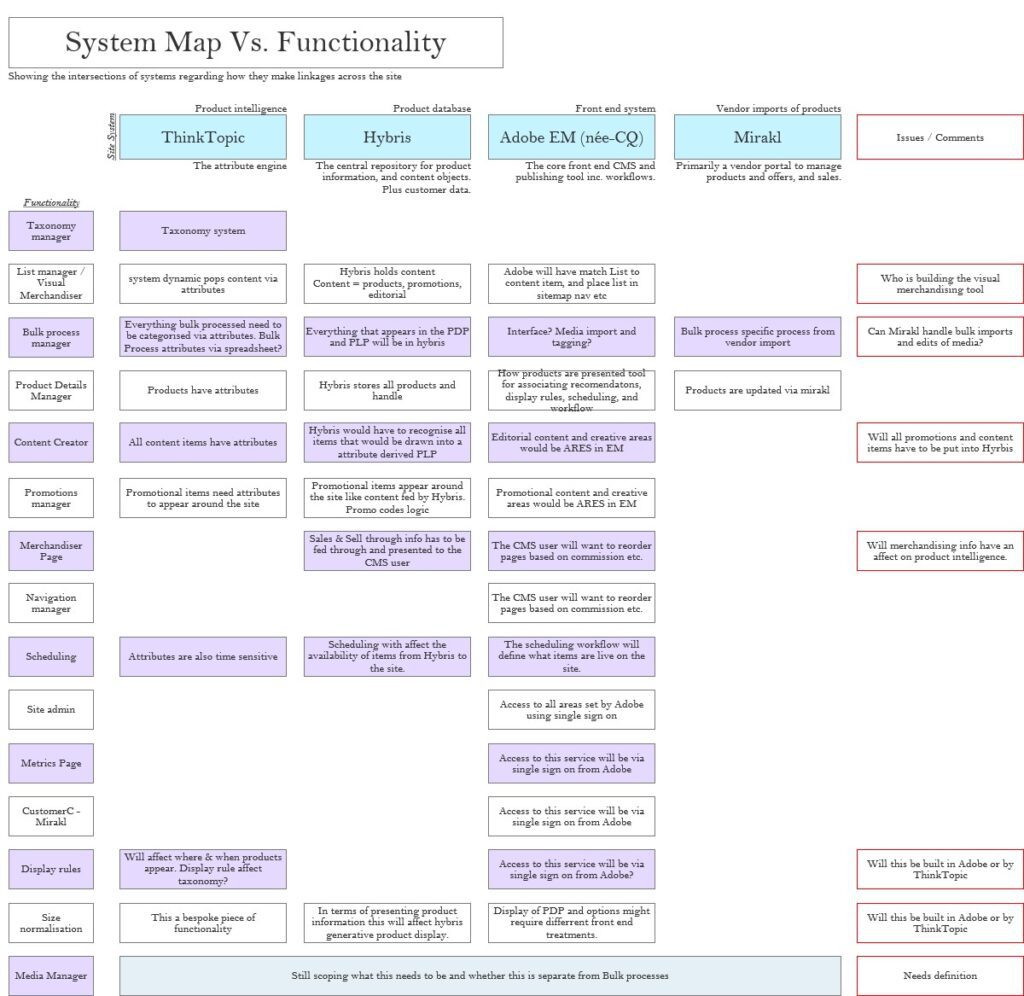
Realisation
“Results matter. Can we validate editorial insight with data and metrics?”
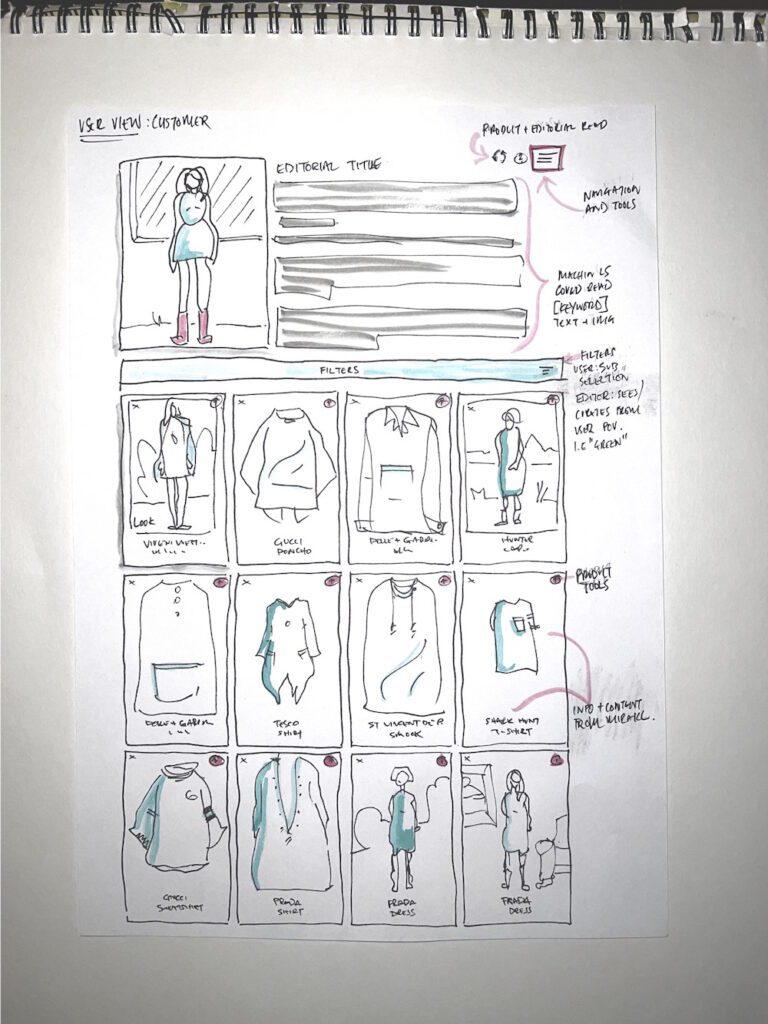
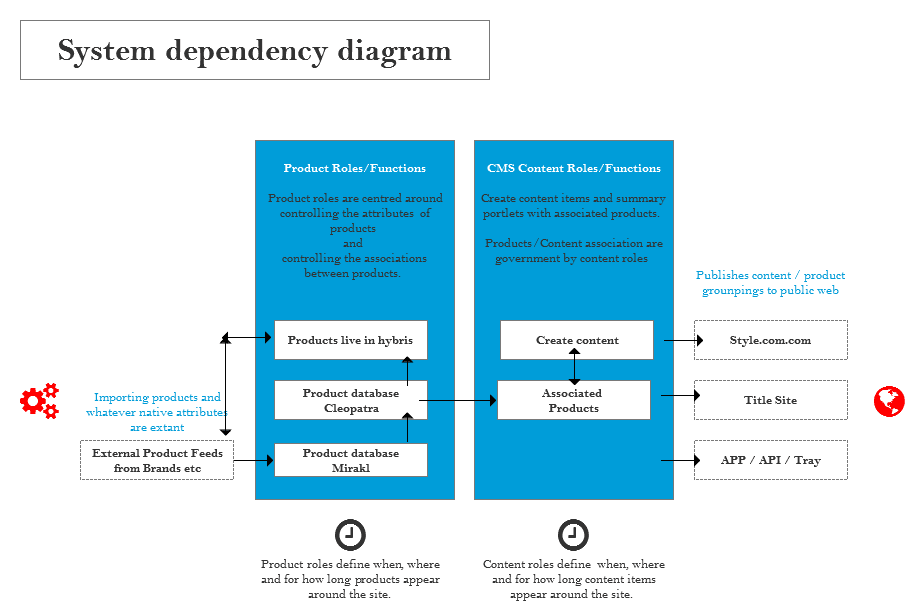
With a workable prototype we were able to iterate the prototype with editors ready for development. We had liaised with the tech team throughout the process (including key people from Google’s DeepMind) to ensure that the CMS would be futureproofed (to some extent) and would be able to make the most of innovations in AI and MLS.
A key ‘backend’ aspect that we never got to see was how MLS could register the actions of users and interpret them as creative choices with aesthetic outputs. From a computational perspective the MLS would have read the editors’ choices as relational and quantitative, and then prioritise that business rule in terms of customer activity and transaction history. A development which would have taken MLS into areas previously considered aesthetic decisions powered by data instead of judgement.
Heady thoughts in 2015.
Activities & Outputs
Pilot strategy and delivery planning, stakeholder presentation, pilot and phase 1 launch agreement.